Why 85% of AI Projects Fail
Artificial Intelligence (AI) holds transformative potential for industries worldwide, driving innovation and efficiency. Yet, a staggering 85% of AI projects fail to deliver on their promises, leaving many organisations to grapple with disappointing outcomes. Understanding why AI projects fail and how to address these pitfalls is crucial for C-level executives looking to harness AI’s full potential.
Why do AI Projects Fail?
According to a Gartner report, 85% of AI projects fail. This alarming statistic underscores the challenges inherent in AI deployment. Key factors contributing to these failures include poor data quality, inadequate data availability, and a limited understanding of AI’s capabilities and requirements (Gartner, 2024). These issues highlight the need for meticulous data management and strategic planning, including the integration of cloud-based models and large language models (LLMs).
The Data Challenge in AI Projects
It’s no secret that data is fundamental to the success of AI and machine learning (ML) initiatives. Without high-quality data, AI models struggle to deliver accurate and reliable results. According to a 2023 McKinsey report, 70% of AI projects fail to meet their goals due to issues with data quality and integration (McKinsey & Company, 2023).
Similarly, Forrester Research’s 2024 study reveals that 68% of organisations face significant data quality and integration challenges that directly impact their AI success (Forrester Research, 2024). Deloitte’s 2024 survey further underscores this issue, with 80% of AI and ML projects encountering difficulties related to data quality and governance, highlighting the critical need for robust data management practices (Deloitte, 2024).
What do these findings tell us? That effective data management and monitoring solutions for achieving successful AI implementations are paramount.
Mastering Data Observability for AI Success
Data is the cornerstone of successful AI and digital transformation projects. Data observability involves a strategic approach to managing data across pipelines to ensure its integrity and performance. Key aspects include:
- Keeping Data Current: Ensures that decision-making is based on the latest information, which is crucial for accurate and timely digital transformation outcomes.
- Monitoring Data Flow: Provides alerts for unexpected changes in data volume, which can reveal underlying issues impacting AI performance and data strategies.
- Spotting Anomalies: Identifies patterns and outliers to detect potential problems early, helping to maintain the integrity of AI applications and digital initiatives.
- Tracking Data Structures: Flags unexpected changes in data schemas to prevent disruptions in AI models.
- Understanding Data Origins: Offers insight into the data’s journey and its impact, facilitating troubleshooting and optimisation.
Our advanced data observability solutions ensure the success of AI projects by maintaining high data quality and relevance throughout digital transformation efforts.
Optimising AI Performance Through Effective Observability
Beyond data, effective AI performance management is crucial. AI observability includes:
- Monitoring Key Metrics: Tracks essential performance indicators like accuracy and efficiency to ensure AI models meet expectations.
- Ensuring Transparency: Provides insights into how AI models make decisions, promoting alignment with business goals.
- Detecting Performance Shifts: Identifies changes in model performance that may indicate issues with accuracy or relevance, enabling timely adjustments.
Enhancing Feedback Loops and Resolving Challenges
- Robust AI observability is supported by effective feedback mechanisms that depend on data observability. Identifying issues such as data drift requires a thorough understanding of data pipelines. By tracing performance discrepancies back to their source, organisations can quickly address issues and maintain reliable AI systems.
Get Help With Your AI Project
We offer state-of-the-art tools for data and AI observability, essential for achieving excellence in AI projects. By providing in-depth monitoring and analysis, we provide practical AI experience to help organisations leverage high-quality data and maintain transparent, reliable AI systems. Maintaining adherence to regulations and governance standards is crucial for AI projects, and we ensure models meet ethical guidelines and regulatory requirements.
Tackling common AI project challenges requires a holistic approach to data and AI observability. Our team is committed to guiding organisations through these complexities, ensuring successful outcomes and transformative results in their digital and AI endeavors.
For more information on how Plan b AI can support your AI initiatives, contact us today here or visit our website www.myplanb.ai.
INSIGHTS
Related Articles
The Top Tech Trends to Watch as We Approach 2025
From improving operational efficiency to enhancing customer experiences, Al has the potential to reshape the entire landscape of banking as we know it.The AI Prescription: How Gen AI is Transforming Healthcare
From improving operational efficiency to enhancing customer experiences, Al has the potential to reshape the entire landscape of banking as we know it.Why 85% of AI Projects Fail
A staggering 85% of AI projects fail to deliver on their promises, leaving many organisations to grapple with disappointing outcomes.
Never miss a story
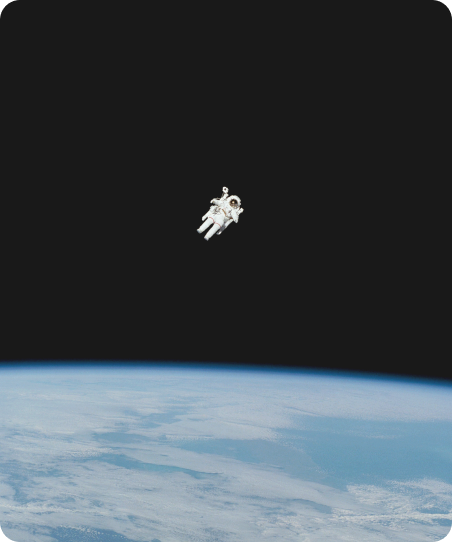